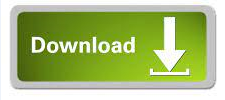
The model represents attention maps with highlighted requirements and information about misclassification to enable cross-dataset evaluations. The model is trained and evaluated on two agricultural datasets that contain plants grown under different conditions: the Plant Seedlings Dataset (PSD) and the Open Plant Phenotyping Dataset (OPPD). Attention maps with an explanation for why a weed is or is not classified as a certain class help agronomists to shape the high-resolution weed identification keys (WIK) that the model perceives. The fusion rule is a weighted average method that is used to combine attention maps from different layers based on saliency. This paper proposes the use of a multi-layer attention procedure based on a transformer combined with a fusion rule to present an interpretation of the DNN decision through a high-resolution attention map. In agriculture, explainable deep neural networks (DNNs) can be used to pinpoint the discriminative part of weeds for an imagery classification task, albeit at a low resolution, to control the weed population.

The result of this study can act as a foundation for the development of an image-based plant recognition system that is able to offer an explanation of a provided classification. The results show that the proposed method can detect the color of flower and labellum very well without having to perform image segmentation. The best overall performance was achieved by the ensemble classifier. In addition, various color scheme scenarios were tested, including the use of primary and secondary color together, and, in addition, the effectiveness of dealing with multi-class classification using multi-class, combined binary, and, finally, ensemble classifiers were studied. As deep learning often excels in pattern recognition in digital images, we applied transfer learning with various amounts of unfreezing of layers with five different neural network architectures (VGG16, Inception, Resnet50, Xception, Nasnet) to determine which architecture and which scheme of transfer learning performs best. It is the first research of its kind using color labels and deep learning for color detection in flower recognition. Using this dataset, a new automated color detection model was developed. In this research, we have built a flower-image dataset, especially regarding orchid species, which consists of human-friendly textual descriptions of features of specific flowers, on the one hand, and digital photographs indicating how a flower looks like, on the other hand. Flower image datasets usually only consist of images and do not contain flower descriptions.

Color labels, such as ‘green’, ‘red’, and ‘yellow’, are used by taxonomists and lay people alike to describe the color of plants. Thus, color is also used in flower-image classification. The color of particular parts of a flower is often employed as one of the features to differentiate between flower types.
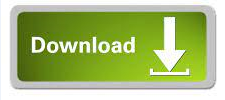